ArkStream Capital track research report: Can AI Agent become the life-saving straw of Web3+AI?
Article source: James ArkStream Capital
TL;DR
The most popular and mature types of AI Agent projects in Web2 startups are mainly enterprise-side services, while in the Web3 field, model training and platform collection projects have become mainstream due to their key role in building an ecosystem.
At present, the number of AI Agent projects in Web3 is small, accounting for 8%, but their market value in the AI track accounts for as high as 23%, so they show strong market competitiveness. We expect that as the technology matures and market recognition increases, there will be multiple projects with a valuation of more than US$1 billion in the future.
For Web3 projects, the introduction of AI technology may become a strategic advantage for non-AI-core application-side products. The combination of AI Agent projects should focus on the construction of the entire ecosystem and the design of token economic models to promote decentralization and network effects.
AI Wave: Current Status of Projects Emerging and Valuations Rising
Since ChatGPT was launched in November 2022, it has attracted more than 100 million users in just two months. By May 2024, ChatGPT's monthly revenue has reached a staggering 20.3 million US dollars. After the release of ChatGPT, OpenAI also quickly launched iterative versions such as GPT-4 and GP4-4o. With such a rapid trend, major traditional technology giants have realized the importance of the application of cutting-edge AI models such as LLM, and have launched their own AI models and applications. For example, Google has released the large language model PaLM2, Meta has launched Llama3, and Chinese companies have launched large models such as Wenxin Yiyan and Zhipu Qingyan. Obviously, the field of AI has become a battleground.
The competition among major technology giants not only promotes the development of commercial applications, but also our survey and statistics on open source AI research show that the 2024 AI Index report shows that the number of AI-related projects on GitHub has surged from 845 in 2011 to about 1.8 million in 2023. In particular, after the release of GPT in 2023, the number of projects increased by 59.3% year-on-year, reflecting the global developer community's enthusiasm for AI research.
The enthusiasm for AI technology is directly reflected in the investment market, which has shown strong growth and explosive growth in the second quarter of 2024. There are 16 AI-related investments of more than US$150 million worldwide, twice as many as in the first quarter. The total financing of AI startups has soared to US$24 billion, more than doubling year-on-year. Among them, Musk's xAI has raised US$6 billion with a valuation of US$24 billion, becoming the second most valuable AI startup after OpenAI.
2024 Q2 AI track financing TOP10, source: Yiou, https://www.iyiou.com/data/202407171072366
The rapid development of AI technology is reshaping the landscape of the technology field at an unprecedented speed. From the fierce competition among technology giants, to the booming development of open source community projects, to the enthusiastic pursuit of AI concepts in the capital market. Projects emerge in an endless stream, investment amounts hit new highs, and valuations rise accordingly. Overall, the AI market is in a golden period of rapid development, and large language models and retrieval-enhanced generation technologies have achieved significant progress in the field of language processing. Despite this, these models still face challenges in converting technical advantages into actual products, such as uncertainty in model output, the risk of generating illusions of inaccurate information, and model transparency issues. These issues become particularly important in application scenarios with extremely high reliability requirements.
Against this background, we began to study AI Agent, because AI Agent emphasizes the comprehensiveness of solving practical problems and interacting with the environment. This shift marks the evolution of AI technology from a pure language model to an intelligent system that can truly understand learning and solve real-world problems. So we see hope in the development of AI Agent, which is gradually bridging the gap between AI technology and practical problem solving. The evolution of AI technology is constantly reshaping the architecture of productivity, while Web3 technology is reconstructing the production relations of the digital economy. When the three elements of AI: data, models, and computing power, are integrated with the core concepts of Web3 such as decentralization, token economy, and smart contracts, we foresee that a series of innovative applications will be born. In this promising cross-field, we believe that AI Agents, with their ability to perform tasks autonomously, have shown great potential for large-scale applications.
To this end, we began to conduct in-depth research on the diverse applications of AI Agents in Web3, from the infrastructure, middleware, and application levels of Web3 to the data and model markets, aiming to identify and evaluate the most promising project types and application scenarios, in order to gain a deeper understanding of the deep integration of AI and Web3.
Concept clarification: Introduction and classification overview of AI Agents
Basic introduction
Before introducing AI Agents, in order to help readers better understand the difference between its definition and the model itself, we use a real-world scenario as an example: suppose you are planning a trip. Traditional large language models provide destination information and travel suggestions. Retrieval-enhanced generation technology can provide richer and more specific destination content. AI Agents are like Jarvis in the Iron Man movie, who can understand needs and actively search for flights and hotels based on your words, perform reservation operations, and add trips to the calendar.
The current industry generally defines AI Agents as intelligent systems that can perceive the environment and take corresponding actions, obtain environmental information through sensors, and affect the environment through actuators after processing (Stuart Russell & Peter Norvig, 2020). We believe that AI Agents are assistants that combine LLM, RAG, memory, task planning, and tool use capabilities. It can not only simply provide information, but also plan, decompose tasks, and actually execute them.
Based on this definition and characteristics, we can find that AI Agent has long been integrated into our lives and applied in different scenarios. For example, AlphaGo, Siri, Tesla's L5 and above autonomous driving can all be regarded as instances of AI Agent. The common feature of these systems is that they can perceive external user input and make corresponding impacts on the real environment accordingly.
Taking ChatGPT as an example to clarify the concept, we should clearly point out that Transformer is the technical architecture that constitutes the AI model, GPT is a model series developed based on this architecture, and GPT-1, GPT-4, and GPT-4o represent versions of the model at different stages of development. ChatGP T is an AI Agent evolved from the GPT model.
Classification Overview
The current AI Agent market has not yet formed a unified classification standard. We have labeled 204 AI Agent projects in the Web2+Web3 market and divided them into primary and secondary classifications according to the significant labels corresponding to each project. Among them, the primary classification is divided into three categories: infrastructure, content generation, and user interaction, and then subdivided according to their actual use cases:
Infrastructure category: This category focuses on building the lower-level content in the Agent field, including platforms, models, data, development tools, and mature B-side services for lower-level applications.
· Development tool category: Provides developers with auxiliary tools and frameworks for building AI Agents.
· Data processing category: Processes and analyzes data in different formats, mainly used to assist decision-making and provide sources for training.
· Model training category: Provides model training services for AI, including reasoning, model establishment, settings, etc.
· B-side service category: Mainly for enterprise users, providing enterprise service, vertical, and automated solutions.
· Platform collection category: A platform that integrates multiple AI Agent services and tools.
Interaction category: Similar to content generation category, the difference is continuous two-way interaction. Interactive Agents not only accept and understand user needs, but also provide feedback through technologies such as natural language processing (NLP) to achieve two-way interaction with users.
· Emotional companionship: AI Agents that provide emotional support and companionship.
· GPT: AI Agents based on the GPT (Generative Pre-trained Transformer) model.
· Search: Agents that focus on search functions and provide more accurate information retrieval.
Content generation: This type of project focuses on creating content, using large model technology to generate various forms of content according to user instructions, and is divided into four categories: text generation, image generation, video generation, and audio generation.
Analysis of the development status of Web2 AI Agents
According to our statistics, the development of AI Agents in the Web2 traditional Internet shows a clear trend of plate concentration. Specifically, about two-thirds of the projects are concentrated in the infrastructure category, mainly B-side services and development tools. We have also conducted some analysis on this phenomenon.
The impact of technology maturity: The reason why infrastructure projects dominate is first of all due to their technological maturity. These projects are usually built on time-tested technologies and frameworks, which reduces the difficulty and risk of development. It is equivalent to a "shovel" in the field of AI, providing a solid foundation for the development and application of AI Agents.
Driven by market demand: Another key factor is market demand. Compared with the consumer market, the enterprise market has a more urgent demand for AI technology, especially in seeking solutions to improve operational efficiency and reduce costs. At the same time, for developers, the cash flow from enterprises is relatively stable, which is conducive to their development of subsequent projects.
Limitations of application scenarios: At the same time, we noticed that the application scenarios of content generation AI in the B-end market are relatively limited. Due to the instability of its output, enterprises prefer applications that can stably improve productivity. This has led to a small proportion of content generation AI in the project library.
This trend reflects the actual considerations of technology maturity, market demand and application scenarios. With the continuous advancement of AI technology and the further clarification of market demand, we expect that this pattern may be adjusted, but infrastructure will still be a solid foundation for the development of AI Agent.
Analysis of leading AI Agent projects in Web2
Collection of leading AI Agent projects in Web2, source: ArkStream project database
We delve into some AI Agent projects in the current Web2 market and analyze them, taking Character AI, Perplexity AI, and Midjourney as examples.
Character AI:
Product Introduction: Character.AI provides AI-based dialogue systems and virtual character creation tools. Its platform allows users to create, train and interact with virtual characters that are able to engage in natural language conversations and perform specific tasks.
Data Analysis: Character.AI had 277 million visits in May and the platform has more than 3.5 million daily active users, most of whom are between 18 and 34 years old, showing the characteristics of a young user group. Character AI has performed well in the capital market, completing a $150 million financing round with a valuation of $1 billion, led by a16z.
Technical Analysis: Character AI has signed a non-exclusive licensing agreement with Google's parent company Alphabet to use its large language model, indicating that Character AI uses self-developed technology. It is worth mentioning that the company's founders Noam Shazeer and Daniel De Freitas were involved in the development of Google's conversational language model Llama.
Perplexity AI:
Product Introduction: Perplexity can crawl and provide detailed answers from the Internet. The reliability and accuracy of information are ensured through citations and reference links. At the same time, it will educate and guide users to ask questions and search for keywords to meet the diverse query needs of users.
Data Analysis: Perplexity has reached 10 million monthly active users, and the number of visits to its mobile and desktop applications increased by 8.6% in February, attracting about 50 million users. In the capital market, Perplexity AI recently announced that it has received $62.7 million in financing, with a valuation of $1.04 billion, led by Daniel Gross, and participants include Stan Druckenmiller and NVIDIA.
Technical Analysis: The main models used by Perplexity are the fine-tuned GPT-3.5, and two large models fine-tuned based on open source large models: pplx-7b-online and pplx-70b-online. The model is suitable for professional academic research and vertical field inquiries, ensuring the authenticity and reliability of information.
Midjourney:
Product Introduction: Users can create images of various styles and themes in Midjourney through Prompts, covering a wide range of creative needs from realism to abstraction. The platform also provides image mixing and editing, allowing users to perform image superposition and style transfer. The platform's real-time generation function ensures that users can get the generated image within tens of seconds to minutes.
Data Analysis: The platform already has 15 million registered users and 1.5 million to 2.5 million active users. At the same time, according to public market information, Midjourney did not take money from investment institutions, relying on the reputation and resources of founder David's multiple entrepreneurial ventures to achieve self-sufficient development.
Technical Analysis: Midjourney uses their own closed-source model. Since the release of Midjourney V4 in August 2022, the platform has been using a diffusion-based generative AI model. The model is said to have 30 to 40 billion training parameters, and this huge amount of parameters provides a solid foundation for the diversity and accuracy of the images it generates.
Commercialization Dilemma
After experiencing multiple Web2 AI Agents, we observed a common path for product iteration: from focusing on a single refined task in the early stage to expanding capabilities to handle more complex multi-task scenarios in the later stage. This trend not only reflects the potential of AI Agents in improving work efficiency and innovation capabilities, but also indicates that they will play a more critical role in the future. Through preliminary statistics of 125 AI Agent projects in Web2, we found that the projects are mainly concentrated in content generation (such as Jasper AI), development tools (such as Replit), and the largest number of B-side services (such as Cresta). This finding is contrary to our expectations. At first, we expected that with the increasing maturity of AI model technology, the C-side market would usher in explosive growth of AI Agent. However, after analysis, we realized that the commercialization path of C-side AI Agent is far more rugged and complicated than expected.
Take Character.Ai as an example. On the one hand, Character.AI has the best traffic performance. However, due to its single business model, it relies on a subscription fee of 9.9USD. In the face of a small amount of subscription income and heavy user reasoning cost consumption, the entire team was eventually acquired by Google due to difficulties in traffic monetization and capital chain problems. This case reflects that even with such good traffic and financing, C-end AI Agent applications are still difficult in the commercialization process. It reflects that the vast majority of products have not yet reached the standard of replacing or effectively assisting manual labor, resulting in C-end users not being willing to pay for current products. In our actual research and investigation, we found that many start-up projects have encountered similar problems as Character.ai. The development of C-end AI Agent is not smooth sailing, but requires more in-depth exploration in technology maturity, product value, and business model innovation to realize its potential and value in the C-end market.
By statistically analyzing the valuations of most AI Agent projects, there is still nearly 10-50 times the valuation space compared to ceiling projects such as OpenAI and xAI. It is undeniable that the ceiling of C-end Agent application is still high enough, proving that it is still a good track. But based on the above analysis, we believe that compared with the C-end, the B-end market may be the final destination of AI Agent. By building a platform, enterprises integrate AI Agent into vertical fields, CRM, office OA and other management software, which not only brings improved operational efficiency to enterprises, but also provides a broader application space for AI Agent. Therefore, we have reason to believe that B-end services will be the main direction of AI Agent development in the traditional Internet of Web2 in the short term.
Analysis of the current status and prospects of Web3 AI Agent development
Project Overview
According to the previous analysis, even AI Agent applications that have obtained top financing and have good user traffic are facing the problem of commercial realization. Next, we will deeply analyze the development of current AI Agent projects in Web3. By evaluating a series of representative projects, including their technological innovation, market performance, user feedback, and development potential, we aim to discover inspiring suggestions. The following figure shows several representative projects with issued tokens and high market capitalization:
Web2's AI Agent leading project compilation, source: ArkStream project database
According to our statistics on the Web3 AI Agent market, the types of project development also show a clear trend of concentrated sectors. Most of the projects are classified as infrastructure projects, and there are relatively few content generation projects. Most projects try to solve the model training needs of the project party by allowing users to provide distributed data, computing power, etc. Or try to build a one-stop platform to embed a variety of AI Agent application services and tools. From development tools to front-end interactive applications, generative applications, etc. The traditional AI Agent industry is currently mainly limited to open source parameter adjustment or applying existing models to build applications. This method has failed to form a significant network effect at the enterprise and individual user levels.
Current Situation Analysis
We believe that this phenomenon may be driven by the following factors at this stage:
Market and technology mismatch: The combination of Web3 and AI Agent currently has no obvious advantages over the traditional market. Its real advantage lies in improving production relations and optimizing resources and collaboration through decentralization. This may lead to some interactive and generative applications lacking competitiveness in front of traditional competitors with stronger technical and financial strength.
Application scenario limitations: In the Web3 environment, there may not be so many practical needs to generate content such as images, videos or text. On the contrary, the decentralized and distributed characteristics of Web3 are more used to achieve cost reduction and efficiency improvement in the traditional AI field, rather than to expand new application scenarios.
The root of this phenomenon, we believe, can be traced back to the current development status of the AI industry and its future direction. It may be because the current AI technology is still in its infancy, similar to the transition period when steam engines were replaced by electric motors in the early days of the Industrial Revolution, and has not yet reached the era of electrification for widespread application.
We have reason to believe that the trend of AI development in the future may follow a similar path. General models will gradually solidify, while fine-tuned models will show diversified development. AI applications will be widely dispersed among various enterprises and individual users, and the focus will shift to the interconnection and interaction between models. This trend is highly consistent with the concept of Web3, because Web3 is known for its composability and permissionless features, which coincides with the concept of decentralized model fine-tuning. Developers are allowed greater freedom to combine and adjust various models more freely. At the same time, the decentralized feature also brings unique advantages to model training in terms of data privacy protection and computing resource allocation.
With technological advances, especially the emergence of new technologies such as LoRA (Low-Rank Adaptation), the cost and technical threshold of model fine-tuning have been greatly reduced. This makes it easier to develop public models for specific scenarios or to meet the personalized needs of users. AI Agent projects within Web3 can make full use of this technological advancement to explore novel training methods, innovative incentive mechanisms, and new models of model sharing and collaboration in the field of model training and fine-tuning, which are often difficult to achieve in traditional centralized systems.
In addition, the concentration of Web3 projects on model training also reflects their strategic considerations of occupying an important position in the entire AI ecosystem. Therefore, AI Agent projects in the Web3 industry are concentrated in the field of model training, which is a natural intersection of technology development trends, market demand and Web3 industry advantages. Next, we will cite several model training projects in the Web2&3 industry and compare them.
Model Training Projects
Humans.ai
Project Introduction: Humans.ai is a diversified AI algorithm model library and training deployment environment covering multiple fields such as images, videos, audio, and text. The platform not only supports developers to further train and optimize models, but also allows them to share and trade their own models. A significant innovation is that Humans.ai uses NFT as a medium to store AI models and user biometric data, making the process of AI content creation more personalized and secure.
Data Analysis: The market value of Humans.ai's token Heart is about $68 million. Twitter followers are 56k, and its user data is not disclosed.
Technical Analysis: Humans.ai does not develop its own models, but adopts a modular approach to encapsulate all provided models into NFTs, providing users with a flexible and scalable AI solution.
FLock.io
Project Introduction: FLock.io is an AI co-creation platform based on federated learning technology (a decentralized machine learning method that emphasizes data privacy). It is committed to solving pain points in the AI track, such as low public participation, insufficient privacy protection, and the monopoly of large companies on AI technology. The platform allows users to contribute data while protecting privacy, promoting the democratization and decentralization of AI technology.
Data Analysis: In early 2024, it completed a $6 million seed round of financing, led by Lightspeed Faction and Tagus Capital, and followed by DCG, OKX Ventures and other institutions.
Technical Analysis: FLock.io's technical architecture is based on federated learning, a method that promotes decentralization while protecting data privacy. In addition, FLock.io also uses technologies such as zkFL, homomorphic encryption, and secure multi-party computing (SMPC) to provide additional protection for data privacy.
This is a model training project for AI Agents in the Web3 industry. There are also similar platforms in Web2 that provide model training services, such as Predibase.
Predibase
Project Introduction: Predibase focuses on AI and large language model optimization, allowing users to fine-tune and deploy open source large language models such as Llama, CodeLlama, Phi, etc. The platform supports a variety of optimization techniques such as quantization, low-rank adaptation, and memory-efficient distributed training.
Data Analysis: Predibase announced the completion of a $12.2 million Series A financing led by Felicis. Large companies such as Uber, Apple, Meta, and startups such as Paradigm and Koble.ai are all users of the platform.
Technical Analysis: Predibase users have trained more than 250 models. The platform currently adopts the LoRAX architecture and Ludwig framework: LoRAX enables users to serve thousands of fine-tuned LLMs on a single GPU, greatly reducing costs without affecting throughput or latency. Ludwig is a declarative framework, and Predibase is used to develop, train, fine-tune, and deploy state-of-the-art deep learning and large language models.
Project Analysis: The Predibase platform is user-friendly and provides customized AI application building services for users at different levels. Whether for C-end and B-end users, or for newcomers or experienced people in the field of AI.
For novices, the one-click automation function of the Predibase platform simplifies the model building and training process. The platform automatically completes complex building and deployment steps. For experienced users, it provides more in-depth customization options. Not only does it provide automation services, but it also allows users to access and adjust more professional parameter settings. When comparing traditional AI model training platforms with AI projects in the Web3 field, although they may be similar in overall framework and logic, we found that there are some significant differences in technical architecture and business model between the two.
Technical Depth and Innovation: Traditional AI model training platforms often adopt deeper technical barriers, such as using self-developed technologies such as LoRAX architecture and Ludwig framework. These frameworks provide powerful functions that enable the platform to handle complex AI model training tasks. However, Web3 projects may focus more on decentralization and openness without in-depth technology.
Flexibility of business model:In the field of traditional AI model training, we have noticed that a common bottleneck is the lack of flexibility in the business model. The platform requires users to pay to train the model, and the source of funds limits the sustainable development space of the project, especially in the early stages that require extensive user participation and data collection. In contrast, Web3 projects will have more flexible business models, such as community-driven models with token economies.
Challenges of privacy protection:Privacy protection is another key issue. Taking Predibase as an example, although it provides virtual private cloud services on AWS, this architecture that relies on third parties always has the potential risk of data leakage.
These points of differentiation have become bottlenecks in the traditional AI industry without exception. Because of the characteristics of the Internet, these problems are destined to be difficult to solve in an efficient way. At the same time, this also brings opportunities and challenges to Web3. The project party that takes the lead in solving these problems will most likely become a pioneer in the industry.
Other categories of Web3 Agent projects
After we explored AI Agent projects for model training, we will expand our vision to other types of AI Agent projects in the Web3 industry. Although these projects are not only focused on model training, they are different in terms of financing data, listing performance, token market value, etc. The following are several AI Agent projects that are representative and influential in their respective fields:
Myshell
Product Introduction: It provides a comprehensive AI Agent platform where users can create, share, and personalize AI agents. These agents can provide companionship and assist work to improve efficiency. The platform covers a variety of AI agent styles, including two-dimensional and traditional styles, and the interactive forms include audio, video, and text. The special thing about MyShell is that it aggregates a variety of existing models including GPT4o, GPT4, and Claude, providing users with a high-level experience of traditional paid AI Agents. In addition, the platform introduces a trading system similar to the FT bonding curve, which encourages creators to develop high-value AI models while giving users the opportunity to invest and share the benefits.
Data Analysis: MyShell's last round of financing was valued at approximately US$80 million, led by Dragonfly, and other well-known investors such as Binance, Hashkey, and Folius also participated. Although there is no specific user access data, MyShell has nearly 180K Twitter followers on social media. Although the number of people online on Discord is usually no more than one-tenth of the total number of fans, it shows that the project has a loyal user and developer base.
Technical analysis: MyShell does not develop AI models independently, but as an integrated platform, it brings together cutting-edge models such as Claude, GPT-4, 4o, and claims to support other closed-source models. This strategy enables MyShell to use existing technical resources to provide users with a unified and advanced AI experience.
Subjective experience: MyShell allows users to freely create and customize AI agents according to their needs, whether as personal companions or professional assistants, and can adapt to a variety of scenarios such as audio and video. Even if users do not use MyShell's agents, they can enjoy the integrated Web2 payment model at a lower cost. In addition, the platform combines the economic concept of FT, allowing users to not only use AI services, but also invest in AI agents they are optimistic about, and increase the wealth effect through the bonding curve mechanism.
Delysium
Product introduction: Delysium provides an intent-centric AI Agent network, allowing Agents to better cooperate to bring users a friendly Web3 experience. At present, Delysium has launched two AI Agents: Lucy and Jerry. Lucy is a networked AI Agent with the vision of being able to provide tool-like assistance, such as querying the Top10 coin holding addresses, etc., but the function of Agent executing on-chain intentions is not yet open, and can only execute some basic instructions, such as staking AGI within the ecosystem or exchanging it for USDT. Jerry is similar to GPT in the Delysium ecosystem, and is mainly responsible for answering questions within the ecosystem, such as token allocation.
Data analysis: In 2022, the first round of fundraising was 4 million US dollars, and in the same year, it announced the completion of a strategic financing of 10 million US dollars. Its token AGI currently has an FDV of about 130 million US dollars. There is no latest user data. According to Delysium's official statistics, as of June 2023, Lucy has accumulated more than 1.4 million independent wallet connections.
Sleepless AI
Product introduction: An emotional companionship game platform that combines Web3 and AI Agent technology, providing virtual companion games HIM and HER, and using AIGC and LLM to immerse users in interactions with virtual characters. During the continuous conversation, users can modify the character's attributes, clothing, etc. Its compatible large language model ensures that the character iterates itself in each conversation and becomes more understanding of the user.
Data analysis: The project has raised a total of US$3.7 million, with investors including Binance Labs, Foresight Ventures and Folius Ventures. The current total market value of the token is about US$400 million. There are 116K followers on Twitter, and according to official statistics, the number of registered reservations has reached 190K, and its active users have reached 43K. It can be said that its user stickiness is still quite strong.
Technical analysis: Although the official has not disclosed which large language model on the market their product is based on, Sleepless AI ensures that users will feel that the character knows them more and more during the chat process. Therefore, when designing LLM training, they train a model for each character separately, and combine the vector database and personality parameter system to give the character memory.
Subjective experience: Sleepless AI uses AI Boyfriend and AI Girlfriend to cut in from the perspective of Free to Play, not just integrating the chat box of the dialogue robot. The project greatly enhances the authenticity of virtual people through high-cost art, continuously iterated language models, high-quality and complete dubbing, and a series of functions such as alarm clocks, sleep aids, menstrual records, and learning companions. Such emotional value is not felt by other applications on the market. In addition, Sleepless AI has created a more long-term and balanced content payment mechanism. Users can choose to sell NFTs without falling into the dilemma of P2E or Ponzi. This model takes into account both the player's income and game experience.
Prospect Analysis
In the Web3 industry, AI Agent projects cover multiple directions such as public chains, data management, privacy protection, social networks, platform services and computing power. From the perspective of token market value, the total token market value of AI Agent projects has reached nearly 3.8 billion US dollars, while the total market value of the entire AI track is close to 16.2 billion US dollars. The market value of AI Agent projects in the AI track accounts for about 23%.
Although there are only about a dozen AI Agent projects, which is relatively small compared to the projects in the entire AI track, their valuation accounts for nearly a quarter of the market. The market value share in the AI track once again verifies our verification that this sub-track has great growth potential.
After the statistics, we raised a core question: What characteristics do Agent projects have to attract excellent financing and be listed on top exchanges? To answer this question, we explored projects that have achieved results in the Agent industry, such as Fetch.ai, Olas Network, SingularityNET and Myshell.
It is not difficult to find that these projects have some significant characteristics in common: They all belong to the platform collection project in the infrastructure category, building a bridge, one end connecting B-end or C-end users with Agent needs, and the other end serving developers and validators - these are users responsible for model debugging and model training. Regardless of the application level, they have established a closed loop of a complete ecosystem.
We noticed that whether the products they provide are on-chain or off-chain related, these do not seem to be the most critical factors. This leads us to a preliminary conclusion: in the field of Web3, the logic of Web2 that emphasizes practical applications may not be fully applicable. For the leading AI Agent products in Web3, building a complete ecosystem and providing diverse functions may be more critical than the quality and performance of a single product. In other words, the success of a project depends not only on what it provides, but also on how it integrates resources, promotes collaboration, and creates network effects within the ecosystem. This ecosystem building ability may be an important factor for AI Agent projects to stand out in the Web3 track.
The correct way for AI Agent projects to integrate in Web3 is not to focus on the deep development of a single application, but to adopt an inclusive model. This approach involves migrating and integrating the diverse product frameworks and product types of the Web2 era into the Web3 environment to build a self-circulating ecosystem. This can also be seen from OpenAI's strategic shift, which chose to launch an application platform this year instead of just updating the model.
In summary, we believe that AI Agent projects should focus on the following aspects:
Ecosystem construction:Go beyond a single application and build an ecosystem with multiple services and functions to promote interaction and value-added between different components.
Token economic model:Design a reasonable token economic model to encourage users to participate in network construction and contribute data and computing power.
Cross-domain integration:Explore the application potential of AI Agent in different fields and create new usage scenarios and value through cross-domain integration.
After summarizing these three aspects, we also provide some forward-looking suggestions for project parties with different focuses. One is for those non-AI core application-side products, and the other is for native projects focusing on the AI Agent track.
For non-AI core application products:
Maintain long-termism, focus on its core products while integrating AI technology, and follow the times and wait for the wind.Under the current technology and market trends, we believe that using AI as a traffic medium to attract users and enhance product competitiveness has become an important means of competitiveness. Although the reality is that how much AI technology actually contributes to the long-term development of the project is still a question mark, we believe that this provides a valuable window for pioneers who dare to adopt AI technology early. Of course, the premise is that they already have a very strong product.
In the long run, if AI technology achieves new breakthroughs in the future, those project parties that have integrated AI will be able to iterate their products more quickly, thereby seizing opportunities and becoming industry leaders. This is just like in the past few years, live streaming on social media platforms has gradually replaced offline sales as a new sales method for traffic export. At that time, those merchants who had excellent products and chose to follow the new trend and try live streaming stood out immediately with the advantage of early intervention when live streaming e-commerce really broke out.
We believe that in the uncertainty of the market, for non-AI core application-side products, considering the timely introduction of AI Agent may be a strategic decision. Not only can it increase the market exposure of the product at present, but it can also bring new growth points to the product in the continuous development of AI technology.
For native projects focusing on AI Agent:
Balancing technological innovation and market demand is the key to success. In native projects of AI Agent, project parties need to focus on market trends, not just technical research and development. At present, some Agent projects in the market that combine Web3 may be too focused on developing in a single technical direction, or have built a grand vision, but product development has failed to keep up. Both extremes are not conducive to the long-term development of the project.
Therefore, we suggest that project parties should pay attention to market dynamics while ensuring product quality, and realize that the AI application logic in the traditional Internet industry is not applicable to Web3. On the contrary, they need to learn from projects that have achieved results in the Web3 market. Pay attention to the labels they have, such as the core functions such as model training and platform collection mentioned in the article, as well as the narratives they have created, such as AI modularization and multi-agent collaboration. Exploring sexy narratives may be the key to a project's breakthrough in the market.
Conclusion Whether it is a non-AI core product or a native AI Agent project, the most important thing is to find the right time and technical path to ensure competitiveness and innovation in a changing market. Project parties should observe market trends, learn from successful cases, and innovate to achieve sustainable development in the market while maintaining product quality.
Summary
At the end of the article, we analyze the Web3 AI Agent track from multiple perspectives:
Capital investment and market attention:Although AI Agent projects in the Web3 industry do not have an advantage in the number of listings, they account for nearly 50% of the market valuation, showing that the capital market highly recognizes this track. With more capital investment and increased market attention, it is a foregone conclusion that more highly valued projects will appear in the AI Agent track.
Competitive landscape and innovation capabilities: The competitive landscape of the AI Agent track in the Web3 industry has not yet been fully formed. At the application level, there is no phenomenal and leading product similar to ChatGPT, which gives new project parties a lot of room for growth and innovation. With the maturity of technology and the innovation of previous projects, the track is expected to develop more competitive products and promote the valuation of the entire track.
Attach importance to token economy and user incentives: The significance of Web3 is to reshape production relations, so that the originally centralized process of deploying and training AI models can be more decentralized. Through reasonable token economic design and user incentive schemes, idle computing power or personal data sets can be redistributed, and data privacy can be protected through solutions such as ZKML, which can further reduce computing power and data costs and allow more individual users to participate in the construction of the AI industry.
In summary, we are optimistic about the AI Agent track. We have reason to believe that there will be multiple projects with valuations exceeding 1 billion US dollars in the AI Agent track. By horizontal comparison, the narrative of AI Agent is sexy enough and the market space is large enough. At present, the market valuation is generally low. Considering the rapid development of AI technology, the growth of market demand, the investment of capital and the innovation potential of enterprises in the track, in the future, as the technology matures and the market recognition increases, this track is expected to have multiple projects with valuations exceeding 1 billion.
References
https://blog.csdn.net/u012842807/article/details/140566345
https://finance.eastmoney.com/a/202407093126222034.html
Russell, S., & Norvig, P. (2020). Artificial Intelligence: A Modern Approach (4th ed.).
This article is from a contribution and does not represent the views of BlockBeats.
Disclaimer: The content of this article solely reflects the author's opinion and does not represent the platform in any capacity. This article is not intended to serve as a reference for making investment decisions.
You may also like
Yesterday, the U.S. Ethereum spot ETF had a net inflow of $52.3 million
A whale who held 12,001 ETH and had been dormant for 8.75 years began to sell it on the chain
Hamster Kombat’s User Base Plummets by 86% Amid Declining Popularity
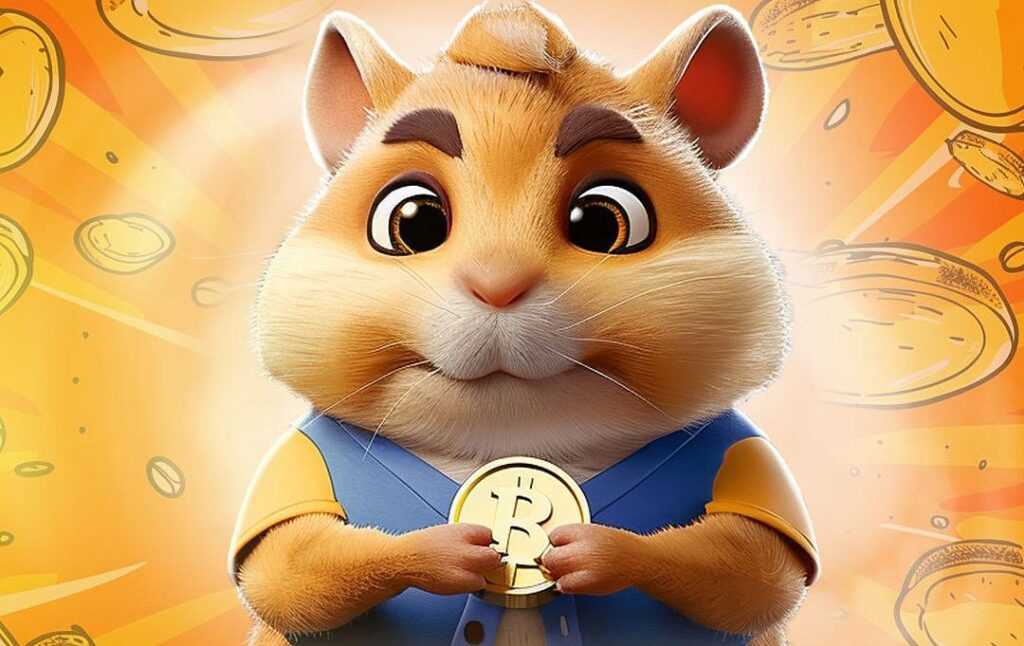
CryptoQuant CEO Issues Warning to Investors Amid Bitcoin Surge
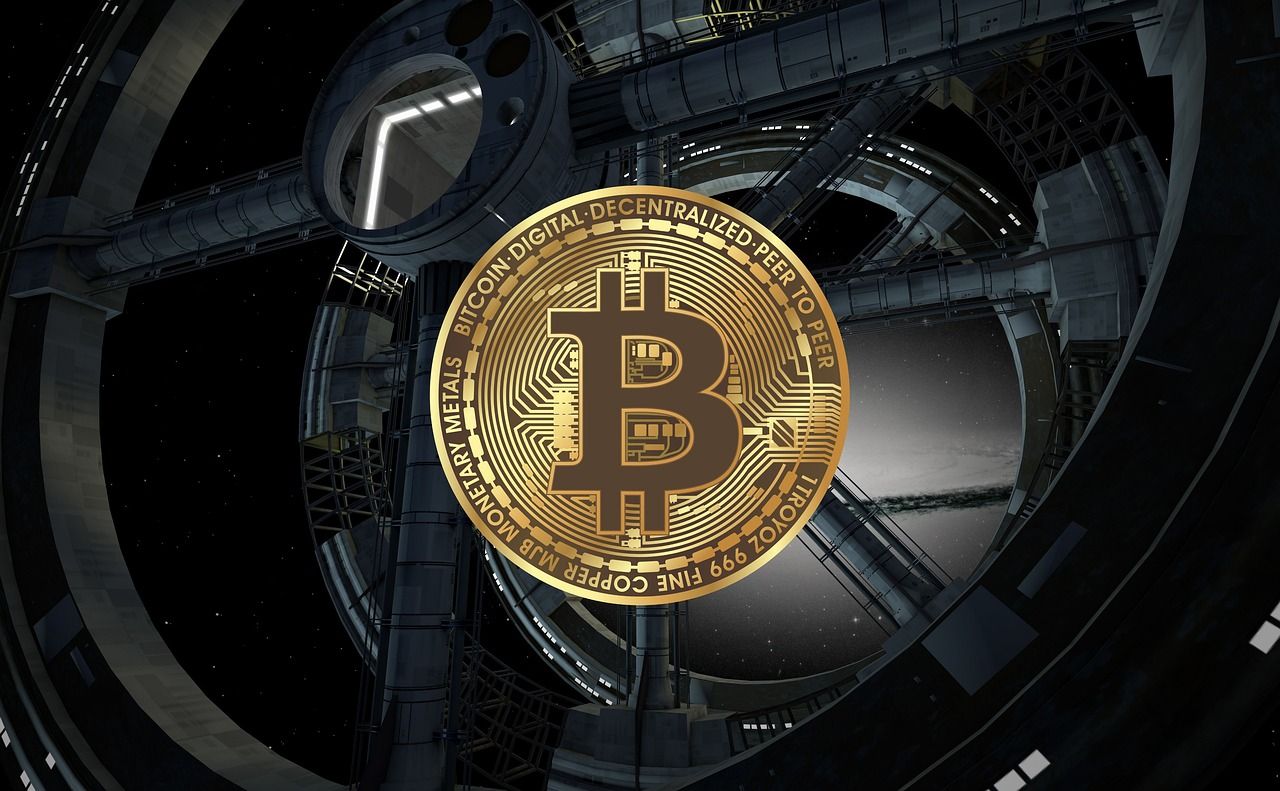
Trending news
MoreCrypto prices
More






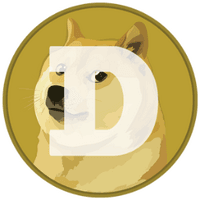

